Approximate Bayesian Inference¶
Below is a quick example of how to use approxposterior
to estimate an
accurate approximation to the posterior distribution of the Rosenbrock Function example from Wang & Li (2018) using the
BAPE algorithm. In many Bayesian inference applications, sampling methods used to derive posterior distributions,
such as Markov Chain Monte Carlo (MCMC) methods, can require >1,000,000 functions evaluations. In cases where the
forward model is computationally expensive, such methods quickly become infeasible. The active learning
approach employed by approxposterior
, however, requires orders of magnitude fewer simulations to
train approxposterior
’s GP, yielding accurate approximate Bayesian posterior distributions.
Note that setting verbose = True also outputs additional diagnostic information, such as when the MCMC finishes, what the estimated burn-in is, and other quantities that are useful for tracking the progress of your code. In this example, we set verbose = False for simplicity.
First, the user must set model parameters.
from approxposterior import approx, gpUtils, likelihood as lh, utility as ut
import numpy as np
# Define algorithm parameters
m0 = 50 # Initial size of training set
m = 20 # Number of new points to find each iteration
nmax = 2 # Maximum number of iterations
bounds = [(-5,5), (-5,5)] # Prior bounds
algorithm = "bape" # Use the Kandasamy et al. (2017) formalism
seed = 57 # RNG seed
np.random.seed(seed)
# emcee MCMC parameters
samplerKwargs = {"nwalkers" : 20} # emcee.EnsembleSampler parameters
mcmcKwargs = {"iterations" : int(2.0e4)} # emcee.EnsembleSampler.run_mcmc parameters
Create an initial training set and gaussian process
# Sample design points from prior
theta = lh.rosenbrockSample(m0)
# Evaluate forward model log likelihood + lnprior for each theta
y = np.zeros(len(theta))
for ii in range(len(theta)):
y[ii] = lh.rosenbrockLnlike(theta[ii]) + lh.rosenbrockLnprior(theta[ii])
# Default GP with an ExpSquaredKernel
gp = gpUtils.defaultGP(theta, y, white_noise=-12)
Initialize the
approxposterior
object.
# Initialize object using the Wang & Li (2018) Rosenbrock function example
ap = approx.ApproxPosterior(theta=theta, # Initial model parameters for inputs
y=y, # Logprobability of each input
gp=gp, # Initialize Gaussian Process
lnprior=lh.rosenbrockLnprior, # logprior function
lnlike=lh.rosenbrockLnlike, # loglikelihood function
priorSample=lh.rosenbrockSample, # Prior sample function
algorithm=algorithm, # bape, agp, jones, or alternate
bounds=bounds) # Parameter bounds
Run!
# Run!
ap.run(m=m, nmax=nmax, estBurnin=True, nGPRestarts=3, mcmcKwargs=mcmcKwargs,
cache=False, samplerKwargs=samplerKwargs, verbose=True, thinChains=False,
onlyLastMCMC=True)
Examine the final posterior distributions
# Check out the final posterior distribution!
import corner
# Load in chain from last iteration
samples = ap.sampler.get_chain(discard=ap.iburns[-1], flat=True, thin=ap.ithins[-1])
# Corner plot!
fig = corner.corner(samples, quantiles=[0.16, 0.5, 0.84], show_titles=True,
scale_hist=True, plot_contours=True)
fig.savefig("finalPosterior.png", bbox_inches="tight")
The final posterior distribution will look something like the following:
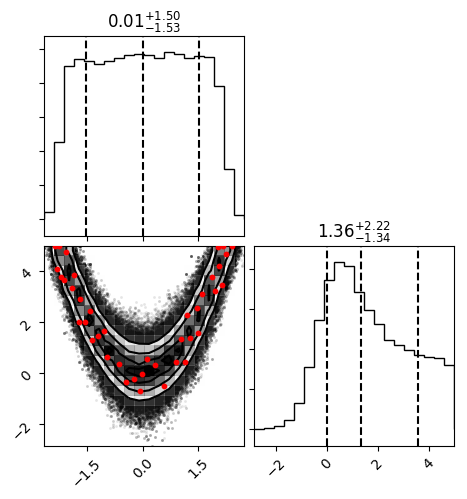
Check the notebook below to see MCMC sampling with using the Rosenbrock function and emcee
.
Jupyter Notebook Examples: