True Rosenbrock Posterior Calculation Using Emcee¶
David P. Fleming, 2019
This notebook demonstrates how to use the Metropolis-Hastings MCMC algorithm to derive the posterior distribution for the Rosenbrock function example presented in Wang & Li (2017) using emcee.
See https://emcee.readthedocs.io/en/stable/ for more information on emcee.
[1]:
%matplotlib inline
from approxposterior import likelihood as lh
from approxposterior import mcmcUtils
import numpy as np
import emcee
import corner
import matplotlib as mpl
import matplotlib.pyplot as plt
mpl.rcParams.update({'font.size': 18})
[2]:
np.random.seed(42)
Set up MCMC initial conditions
[3]:
ndim = 2 # Number of dimensions
nsteps = 20000 # Number of MCMC iterations
nwalk = 20 * ndim # Use 20 walkers per dimension
# Initial guess for walkers (random over prior)
p0 = [lh.rosenbrockSample(1) for j in range(nwalk)]
Initialize MCMC Ensemble Sampler object
Note that here, we use the lh.rosenbrock_lnprob
function that computes the log-likelihood plus the log-prior for the Rosenbrock function model as described in Wang & Li (2017). The functions used to compute this example is provided in approxposterior
. See example.py
in approxposterior
’s examples
directory for to see how approxposterior
’s BAPE algorithm approximates the Rosenbrock function posterior derived in this notebook.
[4]:
sampler = emcee.EnsembleSampler(nwalk, ndim, lh.rosenbrockLnprob)
Run the MCMC!
After the MCMC run finishes, we estimate the burn in following the example in the emcee documentation.
[5]:
sampler.run_mcmc(p0, nsteps)
print("emcee finished!")
emcee finished!
[6]:
# Compute acceptance fraction for each walker
print("Number of iterations: %d" % sampler.iteration)
# Is the length of the chain at least 50 tau?
print("Likely converged if iterations > 50 * (integrated autocorrelation time, tau).")
print("Number of iterations / tau:", sampler.iteration / sampler.get_autocorr_time(tol=0))
Number of iterations: 20000
Likely converged if iterations > 50 * (integrated autocorrelation time, tau).
Number of iterations / tau: [152.45258457 288.91391155]
[7]:
iburn = int(2.0*np.max(sampler.get_autocorr_time(tol=0)))
print(iburn)
262
Let’s visually inspect the chains to see if they’re converged.
The blue dashed line corresponds to our burn in estimate.
[8]:
fig, ax = plt.subplots(nrows=2, sharex=True, figsize=(16,8))
# Plot allllll the walkers and chains
ax[0].plot(sampler.chain[:,:,0].T, '-', color='k', alpha=0.3);
ax[1].plot(sampler.chain[:,:,1].T, '-', color='k', alpha=0.3);
# Plot where the burn in is
ax[0].axvline(iburn, ls="--", color="C0", lw=2.5)
ax[1].axvline(iburn, ls="--", color="C0", lw=2.5)
# Formatting
ax[0].set_xlim(0,nsteps)
ax[1].set_xlim(0,nsteps)
ax[0].set_ylabel("Parameter 0")
ax[1].set_ylabel("Parameter 1")
ax[1].set_xlabel("Iterations")
fig.tight_layout()
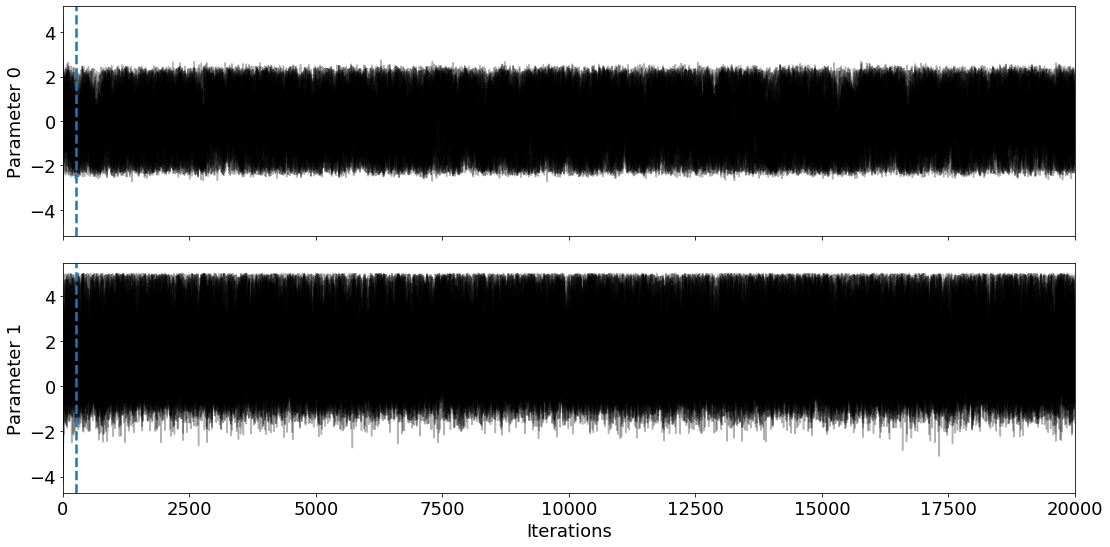
Looks good! Let’s plot the joint and marginal posterior distributions.
[9]:
fig = corner.corner(sampler.get_chain(discard=iburn, flat=True, thin=int(iburn/4.0)),
quantiles=[0.16, 0.5, 0.84],
plot_contours=True, show_titles=True);
fig.savefig("true_post.pdf", bbox_inches="tight")
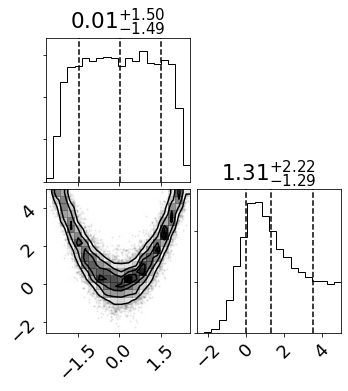
Neat. Now let’s save the sampler object and burn in time into a numpy archive so we can compare our approximate distributions with the truth derived here to see how accurate approxposterior
(in another notebook…)!
[10]:
np.savez("trueRosenbrock.npz",
flatchain=sampler.flatchain,
iburn=iburn)